BACKGROUND ORIENTED SCHLIEREN APPLIED TO STRATIFIED LIQUID CASES
An extension to background oriented Schlieren (BOS) is proposed in the following. The extension enables an accurate reconstruction of the density field in stratified liquid experiments. The multi-media imaging through air-glass-water-glass-air leads to an additional aberration that destroys the reconstruction. A two-step calibration and image remapping transform are the key components that correct the images through the stratified media and provide a non-intrusive full-field density measurements of transparent liquids.
Contents
- LOAD THE IMAGES
- LOAD THE PARAMETERS FILE
- CREATE THE CALIBRATION FIELD: Correlation air-water
- THE REMAPPING
- CORRELATION REFERENCE-REMAPPED
- Comparison between corrected and not-corrected case
- POISSON INTEGRATION
- GRAPHICAL OUTPUT
- ROUTINES
- Parameters.m
- BOS_correlation_OpenPIV.m
- BOS_Remapping.m
- BOS_PoissonSolver.m
- List of the subfunctions contained in BOS_PoissonSolver
- crop_field.m
- create_RHS.m
- CreateGrid.m
- Poisson_equation_2D.m
- Gladstone_Dale.m
LOAD THE IMAGES
We capture and load three images of the background pattern, through air (im1), water (im2) and a saline stratified solution (im3) (im stands for image).
im1=im2double(imread('Data/Air_ref.tif')); im2=im2double(imread('Data/Water_ref.tif')); im3=im2double(imread('Data/4layers.tif'));
LOAD THE PARAMETERS FILE
The parameters file contains: the calibration factor (Mconversion) that has to be measured experimentally since it depends by the camera resolution and distance between camera and backgroud dots; the images size (Lx,Lz) measured in pixels; the boundary coditions (val_up,val_down) involved in the Poisson's integration; the size in pixels (nx_pixel,ny_pixel) of the interrogation area An, used in the PIV cross-correlation algorithm and the overlap of the shifting window (overlap_x,overlap_y) in the two directions x and y.
[Mconversion,Const,Lx,Lz,val_up,val_down,nx_pixel,ny_pixel,overlap_x,...
overlap_y]=Parameters();
CREATE THE CALIBRATION FIELD: Correlation air-water
The calibration is the displacement field $\Delta x$;$\Delta y$ obtained correlating the air and water images (im1,im2).
[Calibration]=BOS_correlation_OpenPIV(im1,im2,nx_pixel,ny_pixel,overlap_x); Magn_cal=sqrt(Calibration.u.^2+Calibration.v.^2); skip = 2; % Skip vectors set(0,'defaulttextinterpreter','latex') figure subplot('position',[0.1 0.1 0.35 0.8]); hold on quiver(Calibration.x(1:skip:end,1:skip:end),Calibration.y(1:skip:end,1:skip:end),... Calibration.u(1:skip:end,1:skip:end),Calibration.v(1:skip:end,1:skip:end),... 'AutoScale','off'); axis equal xlim([min(min(Calibration.x)),max(max(Calibration.x))]) ylim([min(min(Calibration.y)),max(max(Calibration.y))]) title('Calibration vector field') set(gca,'Ydir','reverse') xlabel('x [px]') ylabel('y [px]') subplot('position',[0.55 0.1 0.4 0.8]); contour(Calibration.x,Calibration.y,Magn_cal,50) axis equal xlim([min(min(Calibration.x)),max(max(Calibration.x))]) ylim([min(min(Calibration.y)),max(max(Calibration.y))]) set(gca,'Ydir','reverse') title('Calibration magnitude') xlabel('x [px]') ylabel('y [px]') h = colorbar; ylabel(h,'$\sqrt{\Delta x^{2} +\Delta y^{2}} \hspace{0.25cm}[px]$');
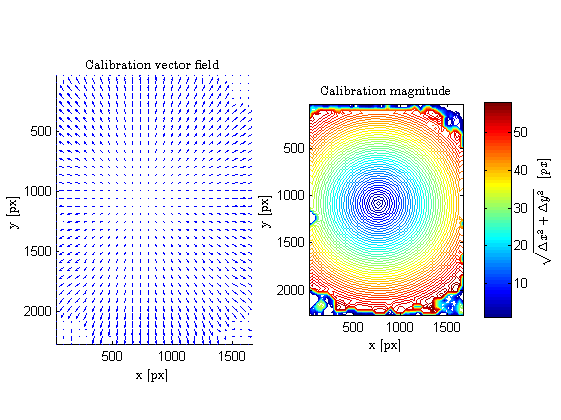
THE REMAPPING
Background pattern image obtained through the saline stratified solution is remapped using the displacement field which origins are in the optical system and aberrations due to the multi-media (air-glass-water-glass-air) imaging
[im3_remapped] = BOS_Remapping(Calibration,im3);
imwrite(im3_remapped,'Remapped_4layers.tif')
CORRELATION REFERENCE-REMAPPED
The corrected image (im3_remapped) is correlated with the original reference image takein in air (im1) and the result is used to construct the Poission equation and to solve it. We suggest to modify size of the An and overlap for a better cross-correlation result since the displacement im1-im3_remapped is one order of magnitude smaller than im1-im2 displ.
nx_pixel=32; ny_pixel=32; overlap_x=0.25; skip = 5; % Skip vectors [Displacement_POisson]=BOS_correlation_OpenPIV(im1,im3_remapped,nx_pixel,... ny_pixel,overlap_x);
Comparison between corrected and not-corrected case
[Displ_notcorr]=BOS_correlation_OpenPIV(im2,im3,nx_pixel,ny_pixel,... overlap_x); figure subplot(121) quiver(Displ_notcorr.x(1:skip:end,1:skip:end),Displ_notcorr.y(1:skip:end,1:skip:end),... Displ_notcorr.u(1:skip:end,1:skip:end),Displ_notcorr.v(1:skip:end,1:skip:end),5); axis equal xlim([min(min(Displ_notcorr.x)),max(max(Displ_notcorr.x))]) ylim([min(min(Displ_notcorr.y)),max(max(Displ_notcorr.y))]) title('Displacement field without correction') set(gca,'Ydir','reverse') subplot(122) quiver(Displacement_POisson.x(1:skip:end,1:skip:end),Displacement_POisson.y(1:skip:end,1:skip:end),... Displacement_POisson.u(1:skip:end,1:skip:end),Displacement_POisson.v(1:skip:end,1:skip:end),5); axis equal xlabel('x [px]') ylabel('y [px]') xlim([min(min(Displacement_POisson.x)),max(max(Displacement_POisson.x))]) ylim([min(min(Displacement_POisson.y)),max(max(Displacement_POisson.y))]) set(gca,'Ydir','reverse') title('Displacement field corrected')
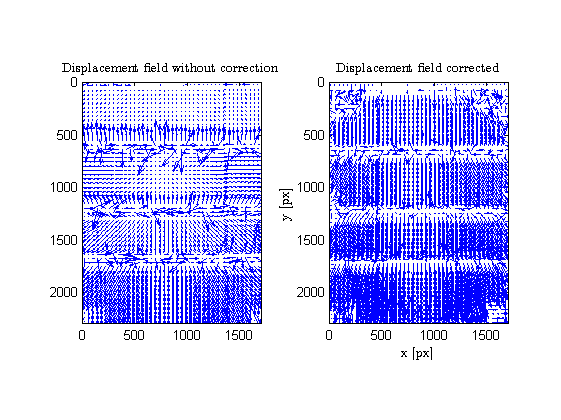
POISSON INTEGRATION
The result of the correlation (im1,im3_remapped) is than integrate through a Poisson's solutor. Eventually by applying the Gladstone-Dale conversion we have computed the 2D density field.
[n2, xc, zc]=BOS_PoissonSolver(Displacement_POisson,Const,Lx,Lz);
[n2_nc, x_nc, z_nc]=BOS_PoissonSolver(Displ_notcorr,Const,Lx,Lz);
% Gladstone-Dale conversion
[Dens,Dens_av]=Gladstone_Dale(n2,xc,zc);
[Dens_2,Dens_av_2]=Gladstone_Dale(n2_nc, x_nc, z_nc);
GRAPHICAL OUTPUT
% ++++++++++++ Comparison Magnitudo +++++++++++++++++++++++++++++++++++++++ Magnitudo=sqrt(Displacement_POisson.u.^2+Displacement_POisson.v.^2); Magnitudo_nc=sqrt(Displ_notcorr.u.^2+Displ_notcorr.v.^2); figure subplot(121) contourf(Displacement_POisson.x*Mconversion,... Displacement_POisson.y*Mconversion,Magnitudo,50) axis equal h1=colorbar; xlabel('x [cm]') ylabel('y [cm]') axis equal xlim([min(min(Displacement_POisson.x*Mconversion)),... max(max(Displacement_POisson.x*Mconversion))]) ylim([min(min(Displacement_POisson.y*Mconversion)),... max(max(Displacement_POisson.y*Mconversion))]) %caxis([0 5]) title('Corrected') set(gca,'Ydir','reverse') ylabel(h1,'$\sqrt{\Delta x^{2} +\Delta y^{2}} \hspace{0.25cm}[px]$'); subplot(122) contourf(Displ_notcorr.x*Mconversion,Displ_notcorr.y*Mconversion,... Magnitudo_nc,50) axis equal h2=colorbar; xlabel('x [cm]') ylabel('y [cm]') axis equal xlim([min(min(Displ_notcorr.x*Mconversion)),... max(max(Displ_notcorr.x*Mconversion))]) ylim([min(min(Displ_notcorr.y*Mconversion)),... max(max(Displ_notcorr.y*Mconversion))]) %caxis([0 5]) title('Not-corrected') set(gca,'Ydir','reverse') ylabel(h2,'$\sqrt{\Delta x^{2} +\Delta y^{2}} \hspace{0.25cm}[px]$'); % ++++ Results: Corrected Magnitude, Density field, Density profiles ++++++ figure subplot('position',[0.08 0.35 0.3 0.4]); contour(Displacement_POisson.x*Mconversion,... Displacement_POisson.y*Mconversion,Magnitudo,20) axis equal h=colorbar; xlabel('x [cm]') ylabel('y [cm]') xlim([min(min(Displacement_POisson.x*Mconversion)),... max(max(Displacement_POisson.x*Mconversion))]) ylim([min(min(Displacement_POisson.y*Mconversion)),... max(max(Displacement_POisson.y*Mconversion))]) % caxis([0 10]) title('Corrected') set(gca,'Ydir','reverse') subplot('position',[0.42 0.35 0.3 0.4]); pcolor(Dens.x*Mconversion, Dens.z*Mconversion, Dens.f') shading flat axis equal tight xlabel('x [cm]') title('Density Corrected') colorbar; set(gca,'Ydir','reverse') subplot('position',[0.77 0.4 0.20 0.45]); hold on plot(Dens_av,Dens.z*Mconversion,'b','linewidth',1.5) plot(Dens_av_2,Dens_2.z*Mconversion,'b--','linewidth',1.5) xlabel('\rho [g/mL]') xlim([0.99 1.3]) ylim([0 28]) Leg=legend('Corrected','Not-Corrected'); set(gca,'Ydir','reverse') set(Leg,'location','northoutside'); % ++++++++++++ Comparison POisson solutions +++++++++++++++++++++++++++++++ figure subplot(121) surf(xc,zc,n2') xlabel('x [px]') ylabel('y [px]') zlabel('n') title('Corrected') subplot(122) surf(x_nc,z_nc,n2_nc') xlabel('x [px]') ylabel('y [px]') zlabel('n') title('Not-corrected')
Warning: Unable to interpret LaTeX string "\rho [g/mL]"
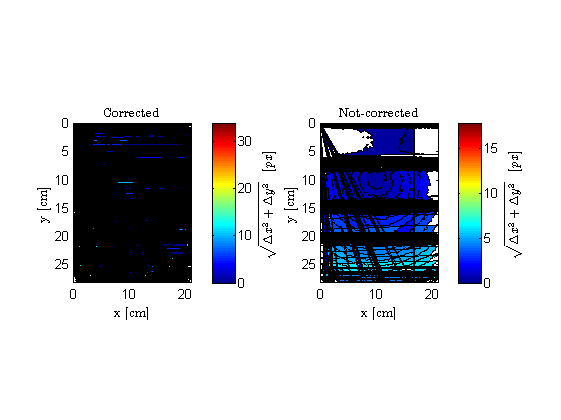
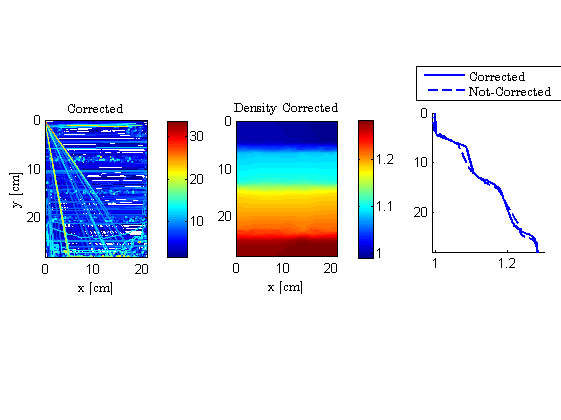
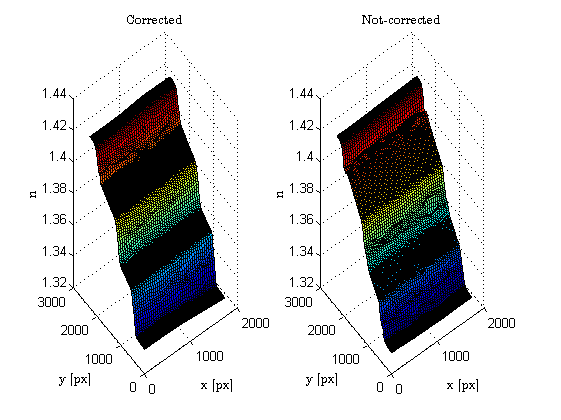
ROUTINES
Parameters.m
PARAMETERS_FILE
% function [Mconversion,Const,Lx,Lz,val_up,val_down,nx_pixel,ny_pixel,... % overlap_x,overlap_y]=Parameters(varargin); % Mconversion=0.0123; % Using the calibration body: pixel/cm % %(see the numbers in the BOS sketch) % B=0*(1/Mconversion); % Distance section 5-6 % W=20*(1/Mconversion); % Distance section 3-4 % L=110*(1/Mconversion); % Distance section 1-2 % t=0.5*(1/Mconversion); % thickness of the glass % ZD=(L+2*t+W+B)*(1/Mconversion); % Set the indexes of refraction % n_air=1; % n_water=1.332; % n_glass=1.43; % Compute the constant of the Poisson equation % Const_inv=2*(((L^2+B^2)/(n_air)) + (t^2/n_glass) + ((W)/(n_water))); % Const=(Const_inv)^-1; % Dirichlet's conditions at the top and bottom % val_up=1.332; % val_down=1.433; % % Size of the images % Lx=1720; % IN PIXEL % Lz=2304; % PIV-parameters % nx_pixel = 64; % ny_pixel = 64; % overlap_x=0.5; % overlap_y=0.5;
BOS_correlation_OpenPIV.m
cross-correlation of two images, using OpenPIV (www.openpiv.net) Inputs: im1,im2 - images Outputs: Displ - displacement field, dx,dy
% function [Displ]=BOS_correlation_OpenPIV(im1,im2,nx,ny,overlap_x); % Add openpiv toolbox: % addpath('./openpiv'); % Overlap in pixels % overlap_px = nx*overlap_x; % pix % overlap_py = nx*overlap_x; % pix % Compute the cross-correlation using OpenPIV % Note that openpiv also saves .VEC file in the image folder if you need % it later % loadvec([imfile1,'.vec']); % [x,y,u,v] = openpiv( im1, im2, ... % nx, ny, ... % overlap_px, overlap_py); % u(isnan(u)) = 0; % v(isnan(v)) = 0; % u = medfilt2(u, [3 3]); % size of the window % v = medfilt2(v, [3 3]); % Displ.x = x; % Displ.y = y; % Displ.u = u; % Displ.v = v;
BOS_Remapping.m
remapping has two steps: 1. interpolate the dx,dy fields to each pixel 2. warp the image according to the interpolated dx,dy [m,n] = size(im1); % note that m is vertical, n is horizontal [X,Y] = meshgrid(0:n-1,0:m-1);
% function [new_image] = BOS_Remapping(Displ,im1); % IMAGE_REMAPPED = BOS_REMAPPING(CALIBRATION,MAGE) % Remaps the second image according to the displacement values stored ... % into the calibration field. % vi = interp2(Displ.x',Displ.y',Displ.v',X,Y,'linear'); % ui = interp2(Displ.x',Displ.y',Displ.u',X,Y,'linear'); % Replace the NaN using zeros % ui(isnan(ui)) = 0; % vi(isnan(vi)) = 0; % im1=flipud(im1); % new_image = flipud(imwarp(im1,ui,vi,true));
BOS_PoissonSolver.m
% function [n2, xc, zc]=BOS_PoissonSolver(Displacement_POisson,Const,Lx,Lz); % Crop the field because of the remapping algorithm % [Displ]=crop_field(Displacement_POisson,Lx,Lz); % If you decide do not to crop the field, uncomment this line % and comment the function crop_field: %Displ=Displacement_POisson; % Create the RHS of the POISSON equation by loading the Displ % function [Rhs]=create_RHS(Displ); % Rotate the RHS % rhs=Const.*fliplr(Rhs); % [Rx,Ry]=size(rhs); % Create the grid % function [xc zc dxb dxc dzc dzb Nx Nz]= CreateGrid(Lx, Lz,Rx,Ry); % Poisson integration % function [n2]=Poisson_equation_2D(Lx,Lz,Rhs,Const);
List of the subfunctions contained in BOS_PoissonSolver
% # crop_field.m % # create_RHS.m % # CreateGrid.m % # Poisson_equation_2D.m
crop_field.m
This function crops the displacement field since the remapping algorithm has created the external frames (lack in the data) due to the moving of the pixels close to the boundary of the image.
% function [Displ]=crop_field(Displacement_POisson,Lx,Lz); % Displ=Displacement_POisson; % Minimum=min(min(Displ.y)); % Displ.y=Displ.y-abs(Minimum); % Magnitude=sqrt(Displ.u.^2+Displ.v.^2); % [a,b]=size(Displ.x); % Dxx=Lx/a; % Dyy=Lz/b; % nx_pixels_crop=700; %250 % ny_pixels_crop=350; %200 % Lx=Lx-nx_pixels_crop; % Lz=Lz-ny_pixels_crop; % % number of pixels to crop in the right and left sides of the image % Dx_pixels=round(nx_pixels_crop/Dxx); % Dy_pixels=round(ny_pixels_crop/Dyy); % Displ.x=Displ.x(Dx_pixels:end-Dx_pixels,Dy_pixels:end-Dy_pixels); % Displ.y=Displ.y(Dx_pixels:end-Dx_pixels,Dy_pixels:end-Dy_pixels); % Displ.u=(Displ.u(Dx_pixels:end-Dx_pixels,Dy_pixels:end-Dy_pixels)); % Displ.v=(Displ.v(Dx_pixels:end-Dx_pixels,Dy_pixels:end-Dy_pixels)); % Magnitude_crop=sqrt(Displ.u.^2+Displ.v.^2); % Displ;
create_RHS.m
The function creates the RHS of the Poisson equation deriving the displacement components obtained correlating the reference with ... the corrected image (im1,im3_remapped).
% function [Rhs,Nx,Nz]=create_RHS(Displ); % u=Displ.u; % w=Displ.v; % x=Displ.x; % z=Displ.y; % [width,height]=size(u); % Compute the central difference fields % Preallocation for the variables du and dw; % du=zeros(width-2,height-2); % dw=zeros(width-2,height-2); % for k=2:width-1 % for j=2:height-1 % du(k,j)=(u(k+1,j)-u(k-1,j))/2*(abs(x(2,2)-x(2,1))); % dw(k,j)=(w(k,j+1)-w(k,j-1))/2*(abs(z(1,1)-z(1,2))); % end % end % Rhs=(du+dw); % Rhs(isnan(Rhs)) = 0 ; % [Nz,Nx]=size(Rhs);
CreateGrid.m
The following function generates the grid where the Poisson eq. will be solved.
% function [xc zc dxb dxc dzc dzb Nx Nz]= CreateGrid(Lx, Lz,Rx,Ry); % Nx=Rx-2; % Nz=Ry-2; % xi=linspace(0,Lx,Nx+1); % xb = xi; % dxc=ones(length(xi)); % dxb=ones(length(xi)); % xc=linspace(0,Lx,Nx+2); % zi=linspace(0,Lz,Nz+1); % zb = zi; % zc=linspace(0,Lz,Nz+2); % dzc=ones(length(zi)); % dzb=ones(length(zi));
Poisson_equation_2D.m
Solving the 2-D Poisson equation by the Finite Difference
...Method. % Numerical scheme used is a second order central difference in space ...(5-point difference). % function [n2]=Poisson_equation_2D(Lx,Lz,Rhs,Const); % [Nx,Nz]=size(Rhs); %Specifying parameters (check why It does not work if we change them) % dx=Lx/(Nx-1); %Width of space step(x) % dy=Lz/(Nz-1); %Width of space step(y) % x=0:dx:Lx; %Range of x(0,2) and specifying the grid points % y=0:dy:Lz; %Range of y(0,2) and specifying the grid points % b=zeros(Nx,Nz); %Preallocating b % pn=zeros(Nx,Nz); %Preallocating pn % Initial Conditions % p=zeros(Nx,Nz); %Preallocating p % Rhs=Const.*fliplr(Rhs); % b=(Rhs); % i=2:Nx-1; % j=2:Nz-1; % Poisson equation solution (iterative) method % tol = 1e-4; % Set tollerance % maxerr = inf; % initial error % iter = 0; % pn=p; % % while maxerr > tol % iter = iter + 1; % disp(['Iteration no. ',num2str(iter)]); %Explicit iterative scheme with C.D in space (5-point difference) % p(i,j)=((dy^2*(pn(i+1,j)+pn(i-1,j)))+(dx^2*(pn(i,j+1)+... % pn(i,j-1)))-(b(i,j)*dx^2*dy*2))/(2*(dx^2+dy^2)); % Boundary conditions % Neumann's conditions % dp/dx|end=dp/dx|end-1 % p(1,:)=p(2,:); % p(end,:)=p(end-1,:); % Neumann's conditions % p(:,1)=p(:,2); % p(:,end)=p(:,end-1); % maxerr = max(max(abs((p-pn)./p))); % disp(['Maximum error is ',num2str(maxerr)]); % pn=p; % end % as long the error larger than tolerance, continue % PG2_gray=p*255; % n_max=1.43; % n_min=1.332; % n2= scaledata(PG2_gray,n_min,n_max);
Gladstone_Dale.m
The function converts the refractive index into density using the Gladstone Dale equation. Galdstone-Dale constant for saline-water can be found here: https://goo.gl/lIwuaL
% function [Dens,Dens_av]=Gladstone_Dale(n2,xc,zc); % G=0.335; %[g/mL] % S_out=(n2-1)./G; % Dens.x=xc; % Dens.z=zc; % Dens.f=S_out; % Dens_av=mean((S_out));